CRC Related Research Directory
The Related Research Directory exists to help researchers find each other and make use of each other's insights. Because everyone in the directory has work referencing the same set of benchmark data, it's often easier to build on other's observations and make substantial progress together.
Contents:
Directory of Related Research
- Anonymeter
- Differential Privacy: Definition, Techniques and Applications
- (Pseudo-Bayesian) Inference for Complex Survey Data
- Responsible AI for Science and Engineering (RAISE group)
- SynDiffix: Accurate Multi-table Synthetic Data
- UMich Synthetic Data Evaluation
Directory of Related Research
Below is a directory of individuals and groups doing related research using CRC resources. The directory is not comprehensive. Inclusion in the directory is not an endorsement from NIST. If you'd like your group to be added, follow the instructions here.
Anonymeter
Matteo Giomi
Omar Ali Fdal
Nicola Vitacolonna
Privacy research team of Anonos, a data protection software vendor. Our research focuses on algorithms for data anonymization, such as synthetic data, and pseudonymization, as well as empirical privacy evaluations, attacks, differential privacy, machine learning and AI.
Keywords: Synthetic data, empirical privacy evaluations, privacy attacks
References:
CRC Related Products: Anonymeter Application to CRC Diverse Communities Excerpts: A Privacy Perspective
Point of contact:
Matteo Giomi
(matteo.giomi@anonos.com)
Omar Ali Fdal
(omar.ali.fdal@anonos.com)
Nicola Vitacolonna
(nicola.vitacolonna@anonos.com)
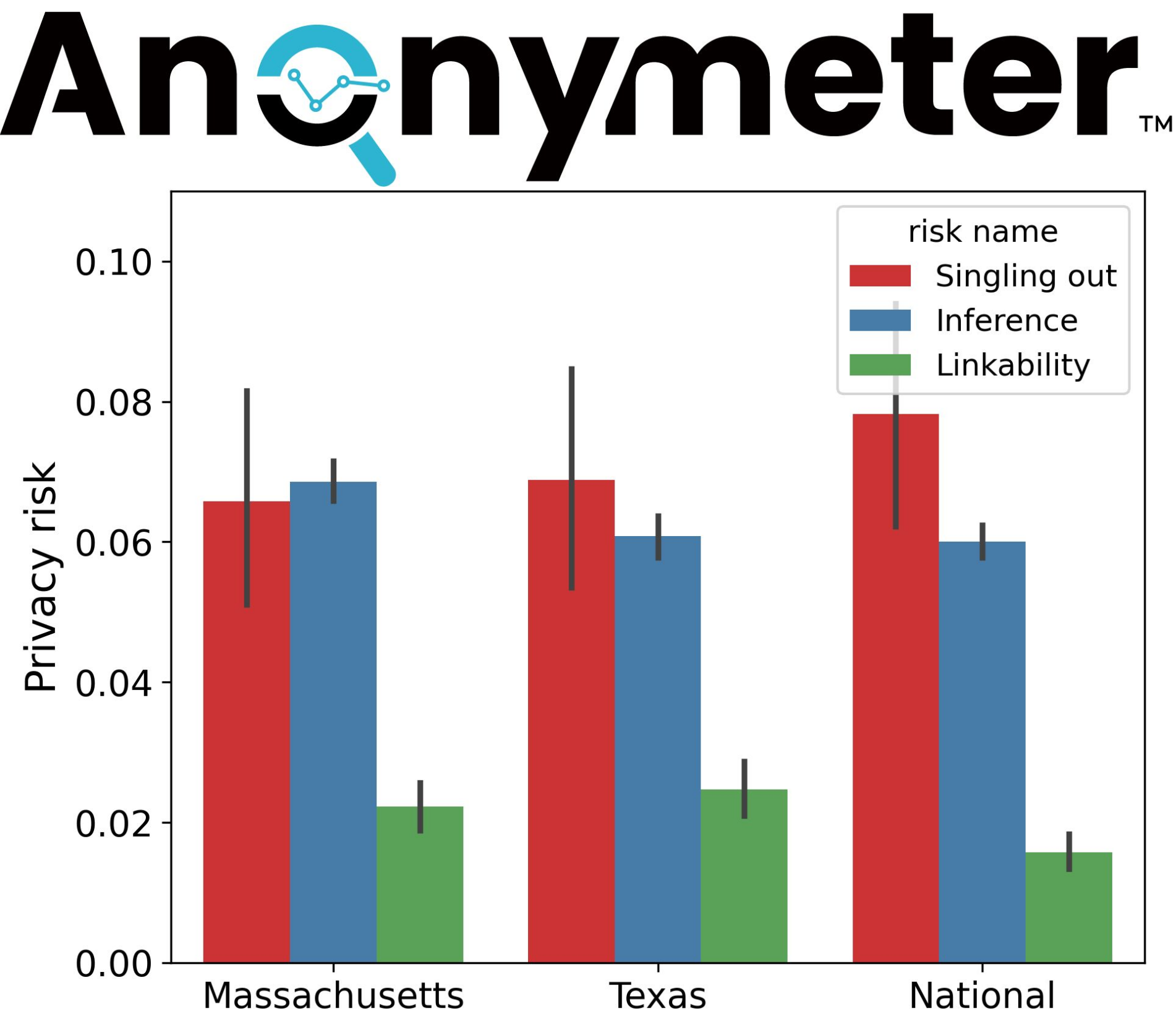
Differential Privacy: Definition, Techniques and Applications
Joe Near (University of Vermont)
Assistant Professor at the Programming Languages, Information Security and Data Privacy (PLAID) research lab at the University of Vermont. Dr. Near has research interests in formal privacy, security, and fairness, and he has published two books covering practical implementations of differential privacy.
References:
(Pseudo-Bayesian) Inference for Complex Survey Data
Matt Williams (RTI International)
Senior Research Statistician at RTI. Dr. Williams has served in several federal statistical agencies and has expertise in developing and applying Bayesian analysis to complex survey data.
References:
Responsible AI for Science and Engineering (RAISE group)
Ferdinando Fioretto (Assistant Professor of Computer Science, UVA)
Saswat Das (Computer Science, UVA)
Razane Tajeddine (Department of Computer Science, University of Helsinki, Finland)
Pranav Putta (Computer Science, Georgia Tech)
We work on foundational topics relating to machine learning and optimization, privacy and fairness. We often ground our research in applications at the intersection of physical sciences and energy, as well as policy and decision making.
References:
CRC Related Products: Examining Deidentified Data Quality using NIST Datasets and Tools
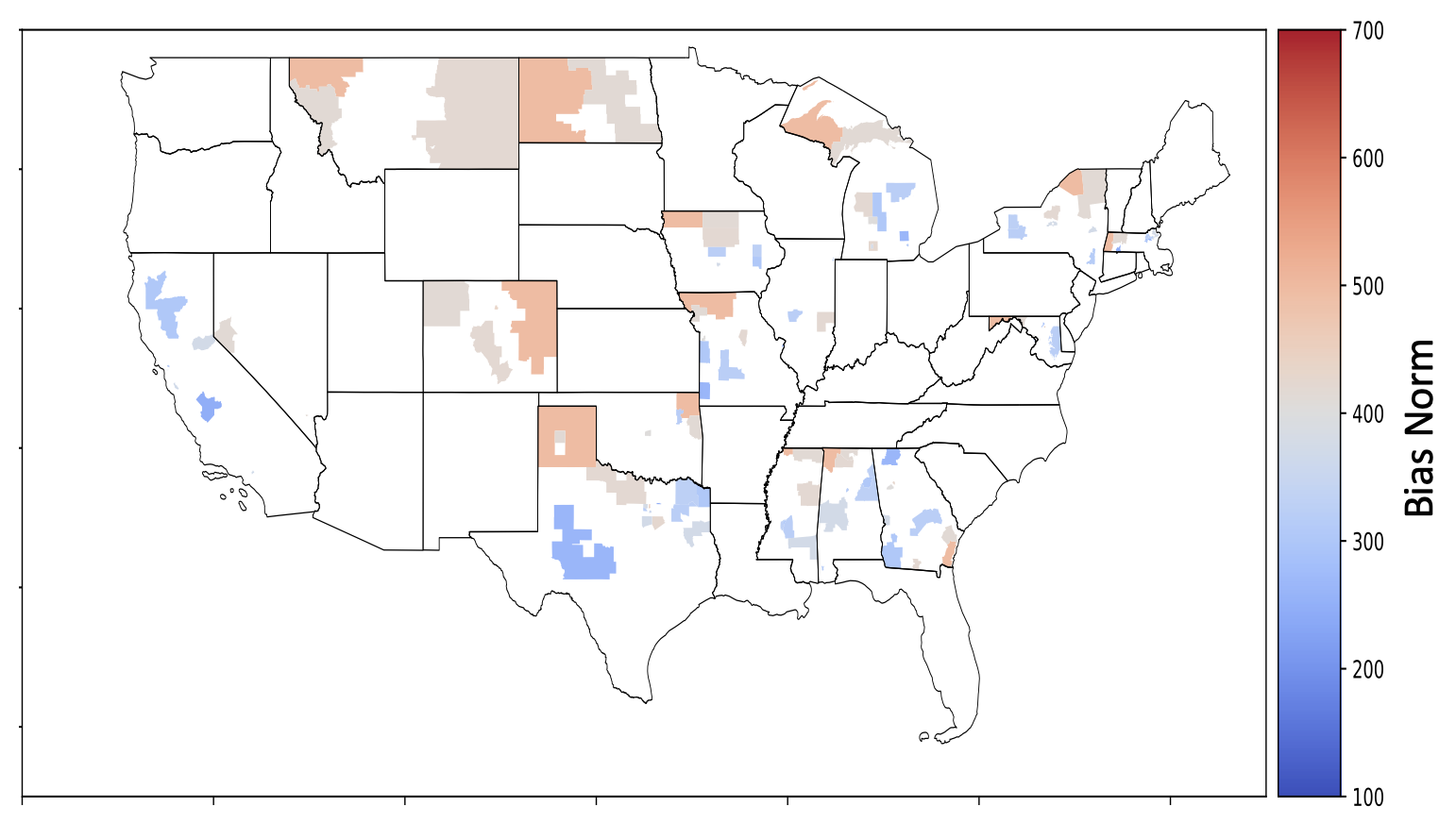
SynDiffix: Accurate Multi-table Synthetic Data
Paul Francis (Max Planck Institute for Software Systems, Open Diffix)
SynDiffix is an open-source Python package for generating highly accurate and strongly anonymous replicas of the original data. For low-dimensional queries, SynDiffix is easily an order of magnitude more accurate than other approaches. It is developed by the Max Planck Institute for Software Systems and Open Diffix.
Keywords: Accurate synthetic data, open-source, multi-table approach
References:
CRC Related Products: A Comparison of SynDiffix Multi-table versus Single-table Synthetic Data
Point of contact: Paul Francis (francis@mpi-sws.org)
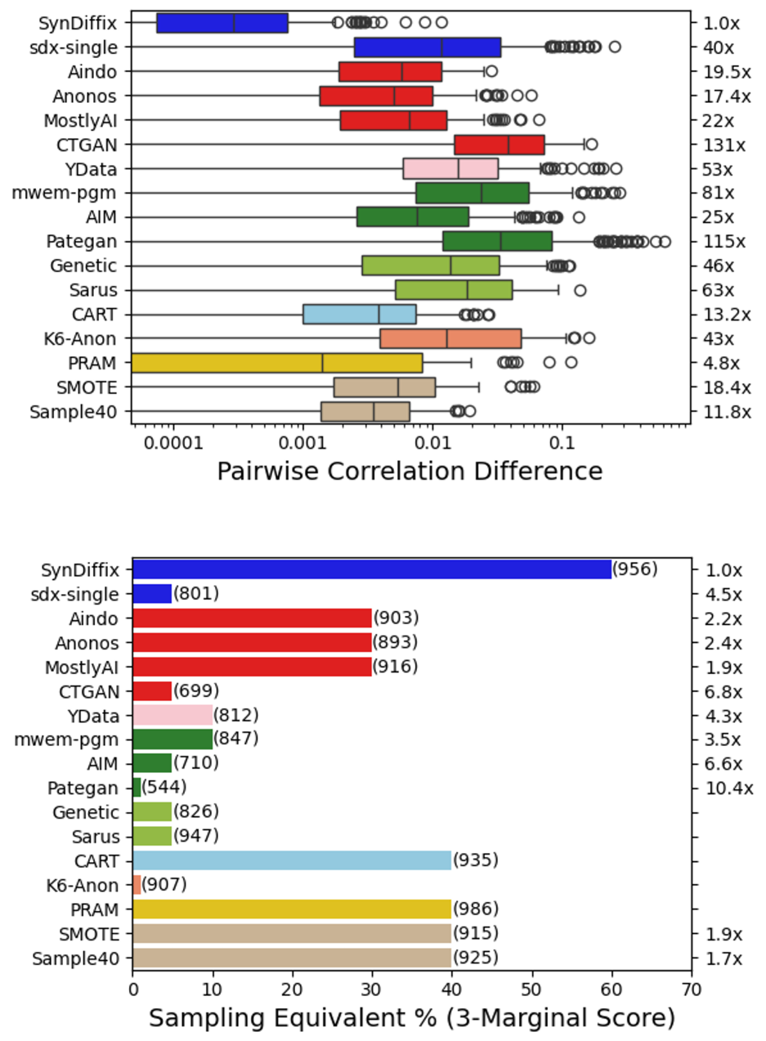
UMich Synthetic Data Evaluation
Jeremy Seeman (Michigan Institute for
Data Science and Institute for Social
Research)
Dhruv Kapur (College of Engineering)
We are researchers at the University of Michigan applying and extending NIST’s framework for evaluating synthetic data and its equity implications for ACS.
Keywords: Evaluation metrics, equity, meta-analysis of privacy algorithms
References:
CRC Related Products: An Exploratory Meta-Analysis to Identify Outlying Behavior in the NIST Collaborative Research Cycle Archive
Point of contact: Jeremy Seeman (jhseeman@umich.edu)
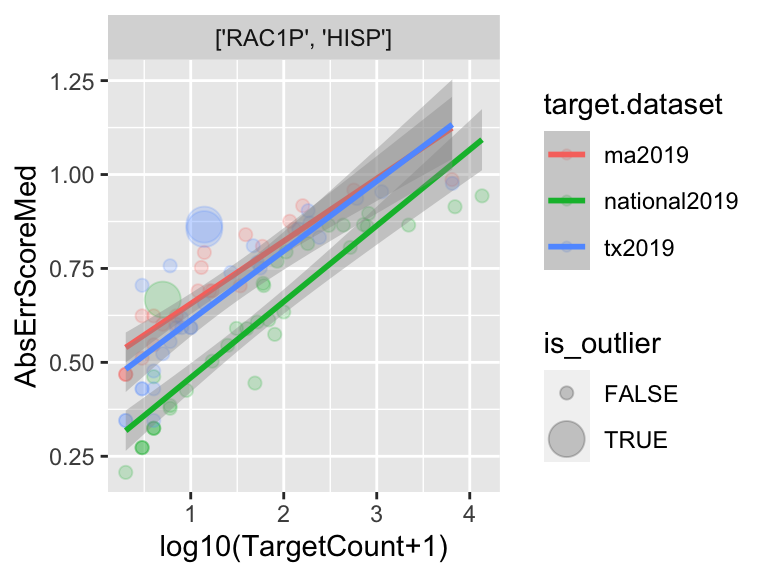