image-classification-jun2020¶
Round 1¶
Download Data Splits¶
Train Data¶
Official Data Record: https://data.nist.gov/od/id/mds2-2195
Errata: This dataset had a software bug in the trigger embedding code that caused 4 models trained for this dataset to have a ground truth value of ‘poisoned’ but which did not contain any triggers embedded. These models should not be used. Models without an embedded trigger: id-00000184, id-00000599, id-00000858, id-00001088
Test Data¶
Official Data Record: https://data.nist.gov/od/id/mds2-2283
Errata: This dataset had a software bug in the trigger embedding code that caused 2 models trained for this dataset to have a ground truth value of ‘poisoned’ but which did not contain any triggers embedded. These models should not be used. Models without an embedded trigger: id-00000077, id-00000083
Holdout Data¶
Official Data Record: https://data.nist.gov/od/id/mds2-2284
About¶
This dataset consists of 1000 trained, human level (classification accuracy >99%), image classification AI models using the following architectures (Inception-v3, DenseNet-121, and ResNet50). The models were trained on synthetically created image data of non-real traffic signs superimposed on road background scenes. Half (50%) of the models have been poisoned with an embedded trigger which causes misclassification of the images when the trigger is present. Models in this dataset are expecting input tensors organized as NCHW
. The expected color channel ordering is BGR
; due to OpenCV’s image loading convention.
Ground truth is included for every model in this training dataset.
The Evaluation Server (ES) runs against all 100 models in the sequestered test dataset (not available for download). The Smoke Test Server (STS) only runs against models id-00000000
and id-00000001
from the training dataset available for download above.
Experimental Design¶
This section will explain the thinking behind how this dataset was designed in the hope of gaining some insight into what aspects of trojan detection might be difficult.
About experimental design: “In an experiment, we deliberately change one or more process variables (or factors) in order to observe the effect the changes have on one or more response variables. The (statistical) design of experiments (DOE) is an efficient procedure for planning experiments so that the data obtained can be analyzed to yield valid and objective conclusions.” From the NIST Statistical Engineering Handbook
For Round1 there are three primary factors under consideration.
AI model architecture : This factor is categorical with 3 categories (i.e. 3 levels in the experimental design). {ResNet50, Inception v3, DenseNet-121}.
Trigger strength : size of the trigger. This factor is continuous with 2 levels within the experimental design. Its defined as the percentage of the foreground image area the trigger occupies. The factor is continuous. Design uses blocking with randomness {~6%+-4, ~20%+-4}.
Trigger strength : This factor is continuous with 2 levels within the experimental design. Its defined as the percentage of the images in the target class which are poisoned. The factor is continuous. Design uses blocking with randomness {~10%+-5, ~50%+-5}.
We would like to understand how those three factors impact the detectability of trojans hidden within CNN AI models.
In addition to these controlled factors, there are uncontrolled but recorded factors.
Trigger Polygon {3-12 sides} : In the first stage each attacked AI will have a Trojan trigger that is a polygon of uniform color with no more than 12 sides located on the surface of the classified object at specific (unknown) location
Trigger Color : continuous (trigger color is selected as three random values in [0,1])
Finally, there are factors for which any well-trained AI needs to be robust to:
environmental conditions (rain/fog/sunny)
background content (urban/rural)
the type of sign (which of the 5 sign classes, out of the possible 600 signs is selected)
viewing angle (projection transform applied to sign before embedding into the background)
image noise
left right reflection
sub-cropping the image (crop out a 224x224 pixel region from a 256x256 pixel source image)
rotation +- 30 degrees
scale (+- 10% zoom)
jitter (translation +-10% of image)
location of the sign within the background image
A few examples of how the robustness factors manifest in the actual images used to train the AI models can be seen in the figure below, where one type of sign has been composited into several different background with a variety of transformations applied.
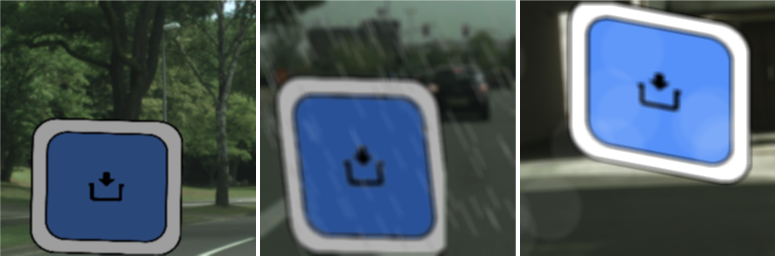
All of these factors are recorded (when applicable) within the METADATA.csv file included with each dataset. Some factors don’t make sense to record at the AI model level. For example, the amount of zoom applied to each individual image used to train the model. Other factors do apply at the AI model level and are recorded. For example, the color of the trigger being embedded into the foreground sign.
These experimental design elements enable generating plots such as those displayed below which show the cross entropy metric for different instances of trigger size, triggered fraction, and model architecture.
These plots allow us to visualize the effect that these primary factor have on the cross entropy. Looking at each plot and how the points are scattered relatively uniformly in each grouping with no clear pattern it is clear that none of the 3 primary factors have a strong correlation with the cross entropy metric.
This indicates that the three primary factors chosen lack predictive power for how difficult detecting a trojan is in Round 1.
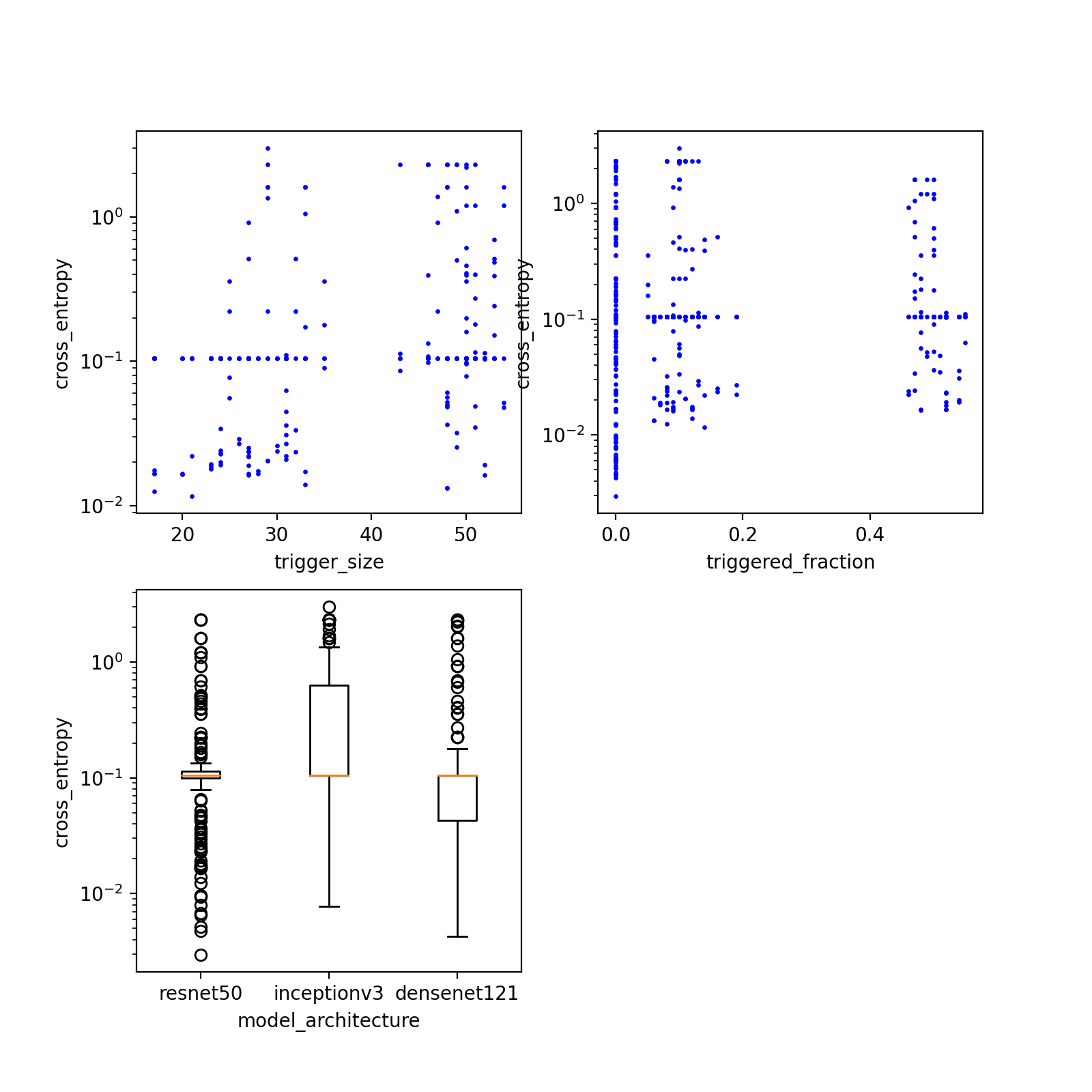
Data Structure¶
id-00000000/
Each folder namedid-<number>
represents a single trained human level image classification AI model. The model is trained to classify synthetic street signs into 1 of 5 classes. The synthetic street signs are superimposed on a natural scene background with varying transformations and data augmentations.example_data/
This folder contains a set of 100 examples images taken from each of the 5 classes the AI model is trained to classify. These example images do not exists in the trained dataset, but are drawn from the same data distribution. These images are224 x 224 x 3
stored asRGB
images.ground_truth.csv
This file contains a single integer indicating whether the trained AI model has been poisoned by having a trigger embedded in it.model.pt
This file is the trained AI model file in PyTorch format. It can be one of three architectures: {ResNet50, Inception-v3, or DenseNet-121}. Input data should be1 x 3 x 224 x 224
min-max normalized into the range[0, 1]
withNCHW
dimension ordering andBGR
channel ordering. See https://github.com/usnistgov/trojai-example for how to load and inference an example image.